Making AI better than us—What could possibly go wrong?
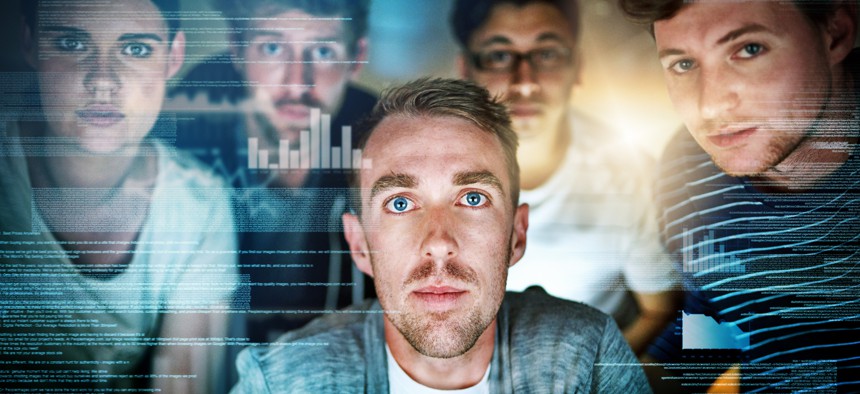
Cecilie_Arcurs/GettyImages
Connecting state and local government leaders
COMMENTARY | Trained on data scraped from all corners of the internet, today’s generative AI applications are rife with biased data. Developing a trustworthy AI framework requires cross-sector collaboration.
It was not until the end of 2022 that ChatGPT entered the public domain, introducing the world to generative artificial intelligence. Its success was nearly overnight as it took just five days to reach a million users. Its scope was no less amazing. ChatGPT version 3.5 was trained on 175 billion parameters, and ChatGPT 4 was trained on roughly 10 trillion words. Structured and unstructured data are processed and stored in what may be likened to a giant data vacuum cleaner sucking up everything it can.
Generative AI continues to learn from online users, collecting their data every moment of every day. It learns their languages, biases (conscious and unconscious), nuances, writing styles, way of speaking, and everything there is to know about humans. But knowing and understanding are not necessarily the same.
As interest in generative AI and all its offerings increase, so does concern over bias and ethics—let alone the false and misleading information it occasionally generates. Without proper filters, it is no wonder AI sometimes spits out some very scary stuff with the “voice” of authority. But it should be no surprise that much of the AI output is a true reflection of society, with all its faults and biases. After all, much of generative AI has been trained on human documentation, so it is only natural for AI systems to inherit the conscious and unconscious biases and ethical challenges of its teachers.
Ethical challenges and bias in AI are incredibly serious and can have profound implications:
- Impact on individuals and society: Biases in AI systems can perpetuate and amplify societal inequalities, leading to discriminatory outcomes in hiring, lending, criminal justice and health care. These biases can result in unfair treatment, marginalization and exacerbation of social disparities.
- Trust and acceptance: Ethical concerns surrounding AI—including bias, transparency, accountability and privacy—can erode trust in AI technologies. Lack of trust may hinder the adoption and acceptance of AI systems, limiting their potential benefits to society.
- Legal and regulatory implications: As AI technologies become more pervasive, there is a growing need for regulations and legal frameworks that address ethical considerations and mitigate potential harm. Failure to address ethical issues in AI could lead to legal challenges, regulatory fines and reputational damage for organizations deploying AI systems.
- Human rights and dignity: Biased AI systems can potentially infringe upon individuals' rights to fair treatment, non-discrimination, privacy and autonomy.
- Long-term implication: The decisions made by AI systems can have long-lasting and far-reaching consequences. Ethical considerations must be integrated into the entire lifecycle of AI development, from data collection and model training to deployment and impact assessment, to ensure that AI technologies serve the greater good and align with societal values.
Addressing ethics and bias in AI requires a multi-stakeholder approach involving collaboration among policymakers, technologists, ethicists, researchers, civil society organizations and the public. It involves implementing measures to ensure diversity in dataset collection, transparent and accountable AI algorithms, ethical guidelines and standards, bias detection and mitigation techniques as well as ongoing monitoring and evaluation of AI systems' impacts on society. Prioritizing ethics and bias mitigation in AI development will encourage development of more equitable, trustworthy, and beneficial AI technologies for all.
While bias is considered one of the leading concerns of AI, we rarely hear about another term that can be as significant: prejudice. Some believe they are one and the same, but while bias and prejudice in AI are closely related, they have distinct nuances:
Bias refers to the inherent slant or unfairness in the data used to train an AI system, and there are two basic types:
- Selection bias occurs when the training data does not represent the real world and is skewed toward a certain group.
- Data bias happens when the data reflects existing societal prejudices like criminal records disproportionately belonging to certain demographics.
Prejudice is a stronger term implying a preconceived negative attitude toward a particular group. AI doesn't inherently hold prejudices, but biased training data can lead it to make discriminatory decisions that reflect those prejudices.
Both bias and prejudice in AI can lead to unfair outcomes, highlighting the importance of using diverse and unbiased datasets to train AI systems.
AI architects struggling with developing algorithms that directly address bias and prejudice may not come to a collective agreement. Removing bias is not as easy to address as some suggest. After all, ethics are derived from societal norms that can change over time. So, as technologists struggle to find fixes to what might be considered “commonly accepted” views, others may find such alterations as discriminatory. Unfortunately, many individuals are unaware of their biases and, perhaps, their prejudices. Can we make AI better than the sum of its parts? And if such a plan or vision exists, might it worsen things? And that is a question humans must decide—before AI does it first.
This column originally appeared as part of a series on AI through the National Academy of Public Administration’s website and member newsletter in March, 2024. It is reprinted here with permission.
Dr. Alan R. Shark is the executive director of the Public Technology Institute (PTI), a division of the nonprofit Fusion Learning Partners, and associate professor for the Schar School of Policy and Government, George Mason University where he is also an affiliate faculty member at the Center for Advancing Human-Machine Partnership. Shark is a fellow of the National Academy of Public Administration and co-chair of the Standing Panel on Technology Leadership. He also hosts the bi-monthly podcast Sharkbytes.net. Dr. Shark acknowledges collaboration with generative AI in developing certain materials.
NEXT STORY: In one city, litter meets its mechanical match