A better way for supercomputers to search large data sets
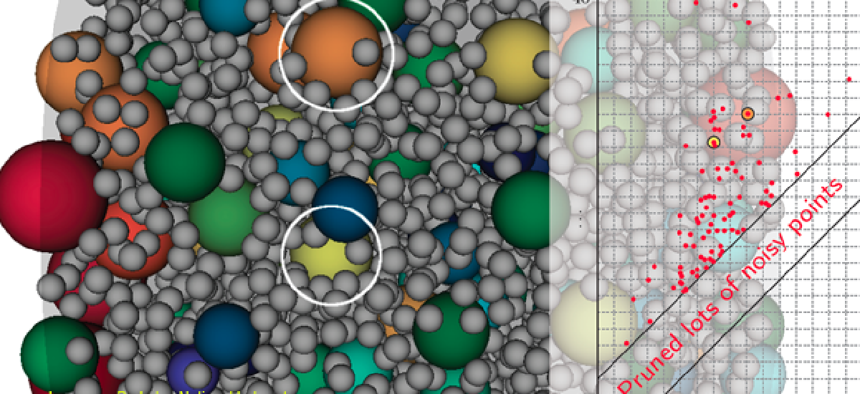
Connecting state and local government leaders
Scientists at Berkeley lab develop "distributed merge trees" that let researchers take advantage of massively parallel architectures to sift through the noise and find what they're looking for.
Scientists are getting better all the time at collecting data, pulling vast amounts of it from expanding networks of sensors, satellite feeds, supercomputers and other devices. The trick is poring over that data and finding useful information, and even with the big data analysis tools at hand, that’s not an easy task.
But researchers at Lawrence Berkeley National Lab may have just made the job easier.
A team at the Energy Department lab has developed new techniques for analyzing huge data sets, by using an approach called “distributed merge trees” that takes better advantage of supercomputing’s massively parallel architectures, according to a report from the National Energy Research Scientific Computing Center.
With supercomputing being applied to everything from genomics to climate research, data sets are getting more complex as well as increasingly large, often running into the petabyte range. Their complexity often puts them beyond the range of standard methods of creating a topology, which has led scientists to apply massively parallel supercomputing techniques during analysis.
But even supercomputers can run up against their limits. “The growth of serial computational power has stalled, so data analysis is becoming increasingly dependent on massively parallel machines,” Gunther Weber, a computational researcher in Berkeley Lab’s Visualization Group, said in the report. “To satisfy the computational demand created by complex data sets, algorithms need to effectively use these parallel computer architectures.”
And that’s where the distributed merge tree algorithms come in. They’re capable of scanning a huge data set, tagging the values a researcher is looking for and creating a topological map of the data, the way a pocket map of the London subway cleanly depicts what is a vast labyrinth of tracks, tunnels and stations.
Distributed merge trees essentially divide and conquer the large topological data sets, separate the data sets into blocks and leverage a supercomputer’s massively parallel architecture to distribute the work across its thousands of nodes, Weber said. In the process, it separates important data from the “noise,” or irrelevant data, inherent in any large data set, the researchers said.
An example would be a topological map of the fuel consumption values within a flame, said Dimiti Morozov, who co-authored a paper on distributed merge trees with Weber. The algorithm would “allow scientists to quickly pick out the burning and non-burning regions from an ocean of ‘noisy’ data,” he said.
Ultimately, distributed merge trees will let scientists get more out of the future supercomputers. “This is also an important step in making topological analysis available on massively parallel, distributed memory architectures," Weber said.