GeoMesa tames big data for GIS in the cloud
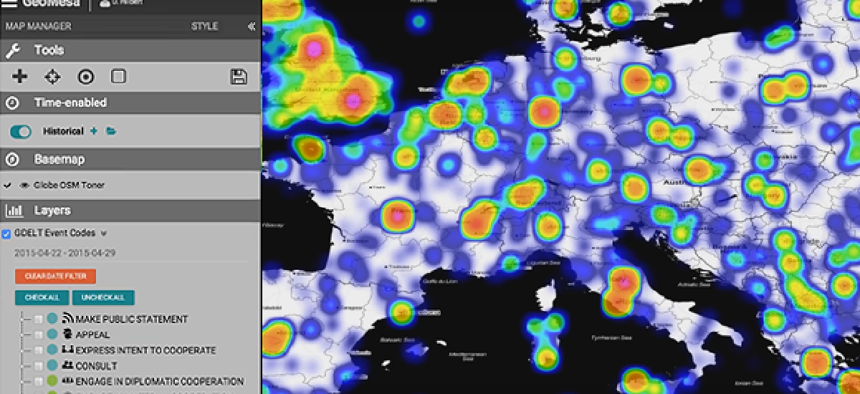
GeoMesa distributes geospatial data processing over the scalable resources of Hadoop, virtually eliminating the limits to the amount of data that can be managed.
Whether it’s for weather or traffic analysis -- or tracking troop movements and terrorists -- geographic information systems have increasingly become the tool of choice for analyzing massive streams of location-sensitive data. As the flow of data to be processed has mushroomed, however, the ability of desktop and server-based GIS to keep up is often exceeded.
That’s the problem developers at CCRi, a Charlottesville, Va.-based analytics firm, faced when working with the Army on creating an algorithm to predict threats from improvised explosive devices in Iraq.
In late 2009, the Army started moving to the cloud with development of its Distributed Standard Cloud. “We started to build our analytics in the cloud as part of the DSC, and we realized that these cloud databases had none of the spatial GIS support at the data layer that we were used to,” James Conklin, director of operations at CCRi, said. The company had previously used PostGIS, a spatial database tool that allows location queries to be run in SQL, but “when we got to the cloud there was no PostGIS,” he said. "In fact, there were no relational databases at all. So we had to figure out how to do the spatial processing in the cloud.”
As a result, CCRi, with the help of the open-source organization Eclipse Foundation and its LocationTech working group, developed GeoMesa, an open-source tool for processing streams of geospatial data on Apache Hadoop. By distributing the data processing over the scalable resources of Hadoop, there’s virtually no limit to the data that can be managed.
“When you start talking about billions or even hundreds of billions of points you’re just going to scale past what you can put on a single server,” Conklin said.
Part of the solution of distributed processing of massive amounts of geospatial data, he said, is reliance on column-family databases. Unlike typical SQL databases, which organize data in rows, column-family databases organize data in sets of columns, a structure that is more efficient for indexing and distributing data across multiple nodes.
GeoMesa currently works with three major Hadoop-based database systems: Apache Accumulo, Apache HBase and Google Cloud Bigtable. And GeoMesa also offers a streaming datastore based on Apache Kafka, a distributed messaging system that provides a fast, high-throughput platform for handling real-time data feeds. With Kafka, GeoMesa can make selected data available in near real time, while shunting other data for further processing.
“Because we’ve had to support high-velocity, high-volume streaming data -- and have near-real-time requirements from a number of our customers -- we have developed a Kafka datastore as well,” Conklin said. “Data that is coming in and needed near real time goes into a Kafka queue, which we make available so that you can show a common operational picture map of the current situation in your real time.” Additionally, he said, data can be sent “to GeoMesa in Accumulo for further analysis.”
“After we got it all working,” said Conklin said, “we realized, ‘Hey, this is not only valuable to us, but this is inherently a very valuable capability.' So we decided to open source it as GeoMesa.”
That decision initiated a process of vetting by LocationTech to ensure that the program meets all geospatial standards. According to Conklin, version 1.2 of GeoMesa, which was released last month, marks the end of the incubation period. “It’s now considered a mature, open source project,” he said.
According to Conklin, the team is also working to add support for Cassandra and Dynamo DB. “Basically, we’re creating versions of GeoMesa that can sit on all of the major big data column-family databases,” he said.
As of now, GeoMesa’s user base is primarily federal, though Conklin said that telecom companies and the auto industry -- which also are working on applications that will stream massive amounts of geospatial data -- are showing interest in adopting it.
“I would’ve thought that [commercial geospatial companies] would have wanted to move in this direction six years ago,” Conklin said. “I think they’ve been very slow to move to the cloud, and there is no commercial equivalent that runs using a cloud distributed database. I’m proud that we’ve gotten this out and made an open source package that will give them a run for their money.”
According to Mansour Raad, senior software architect and big data advocate at Esri, the Redlands, Calif.-based GIS company, his company has not neglected the cloud or distributed data storage and processing of geospatial data. Noting that many federal clients use Accumulo, thanks to its cell-level security, Raad said Esri developers use GeoMesa as a bridge between their Hadoop/Accumulo platforms and ArcGIS applications.
“We’re taking advantage of the Hadoop infrastructure to submit jobs that will do massive, massive work, and then we can take the results back and visualize it as results on the map,” Raad said. “Once there, we can do further geoprocessing on the data for geostatistical significance, as an example.”
While Esri expects increasing use of the cloud for geospatial data storage and processing, Raad noted that Hadoop is not the only platform on which to do cloud-based data analytics, nor is it the only platform that supports distributed storage and processing of geospatial data. Esri supports users employing Hadoop, he said, but the company has decided to focus on developing its own platform for geoanalytics and real-time analytics for more than Hadoop.
And, Raad added, platforms that natively support geospatial data are being updated to implement cell-level security, which will make them more attractive to security-conscious enterprises in the private sector, such as health-care companies.