Deep learning quickly finds structures affected by lava
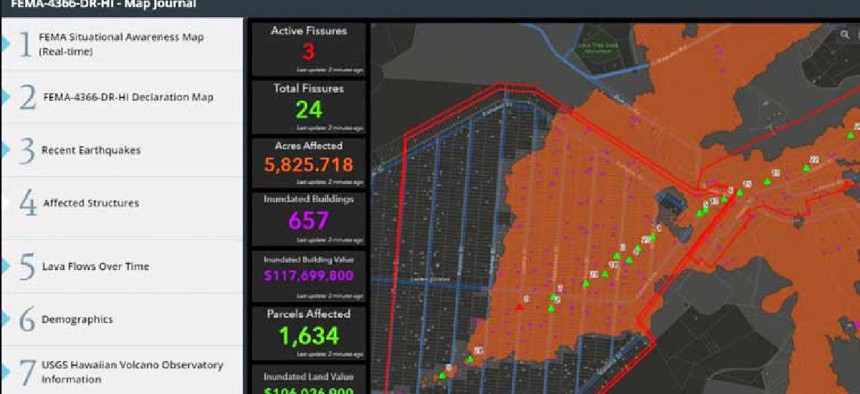
Connecting state and local government leaders
The Federal Emergency Management Agency is using data from Oak Ridge National Lab to see what structures have been overtaken by the Kilauea lava flow.
The Kilauea volcano in Hawaii began erupting in early May and has been doing so continuously since May 27, spewing lava across the Big Island and damaging more than 700 homes. Although there is a chance it could slow down or stop, it's more likely that the flow will continue, according to a new report from the U.S. Geological Survey's Hawaiian Volcano Observatory.
The lava from Kilauea has already had a devastating impact on residences, businesses, schools and hospitals. Officials at the Federal Emergency Management Agency have been getting insight into the buildings and infrastructure affected by the stream of molten rock with the help of Oak Ridge National Laboratory in Tennessee.
By analyzing satellite images of the area around the volcano and combining that information with property ownership data, officials can determine which buildings have been damaged and whether people are evacuating ahead of the lava's approach, said Mark Tuttle, a project developer at ORNL.
FEMA reached out to the lab on May 9 because it had information on parcel locations but not building footprints, said Chris Vaughan, a geospatial information officer at FEMA. From satellite imagery, “Oak Ridge National Laboratory staff were able to extract building footprints for structures, at first starting around the Leilani Estates and Lanipuna Gardens area within the first week and then for most of the Big Island within two weeks,” Vaughan added.
ORNL receives its satellite images from DigitalGlobe, a satellite imaging and analysis company. Researchers then sharpen and adjust the images for perspective and select segments to help train a convolutional neural network that allows them to rapidly extract information from the images about buildings and other structures.
The number of segments needed to train a neural network depends on the size of the area researchers want to model and the diversity of the landscape within that area. Before deep learning, the process was done with basic segmentation, but it was difficult for researchers to generalize the data to a larger area, said Melanie Laverdiere, a research scientist at ORNL.
“With deep learning, we now have the power to create one model for a county or a state or a region, something that can be generalizable to a large geographic area,” she added.
For FEMA's Hawaii project, however, the lab didn’t have to create a model -- the final product of a trained neural network -- because it already had one that would work. “We had a model we had developed in a [different] tropical region, and we were able to apply that to Hawaii,” Laverdiere said.
After the training segments are identified, the model can be run, and the structures in a given area can be pieced together.
ORNL’s supercomputing power allows researchers to work with massive amounts of satellite data, Tuttle said. The FEMA project is currently running on the Titan supercomputer.
“As an example, we processed the entire country of Yemen on Titan in less than two hours,” Laverdiere said. “If we used some of our other computer resources, it could take days [or] weeks.”
Nevertheless, the researchers plan to apply for time on the new Summit machine, which will give them even more storage and computing power. The increased capabilities will mean quicker results.
As ORNL researchers run the model and find the structures in a given area, they send the data to FEMA, which uses Esri's GIS tools to combine it with information on the location of the lava and evacuation boundaries.
“The data gave us a rough estimate of how many structures were presumed destroyed by lava and served as our initial base for finding potential [disaster assistance] recipients,” Vaughan said. By layering the building data on top of information about the lava flow, FEMA was "able to produce the analysis necessary to estimate the number of structures affected and, with parcel data, get a rough estimate of land and building value of affected structures.”
FEMA also combined the data with Hawaii County’s information about road closures so analysts could see properties that weren't “affected by lava flow but were cut off from any potential route to access that structure,” Vaughan said.
After last year's hurricanes, ORNL scientists used the technology to help response teams assess properties damaged by the storms. The ability to extract building outlines and roadways from satellite images ahead of the storms' approach was crucial to recovery efforts because floodwaters tend to obscure and modify structures.
ORNL plans to create a library of models that can find structures in any location in the world. The lab has 10 to 15 models in production and has developed hundreds more. There are also plans to add machine-learning capabilities so the models can identify the types of structures they are finding.
The ability to pinpoint what a structure is would “add greater value to what we’re trying to accomplish,” Tuttle said.