How AI helps predict fire risk
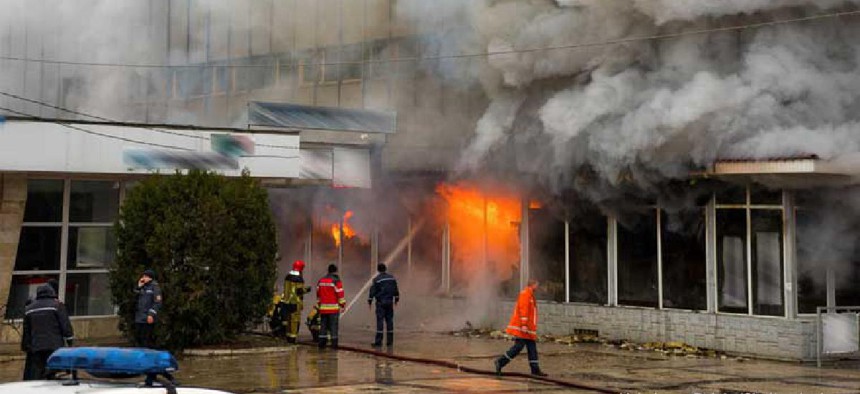
A machine-learning-based model helps the Pittsburgh Bureau of Fire see which buildings in the city are at the highest risk of a fire and prioritize safety inspections.
With a glance at a dashboard and interactive map, Pittsburgh Bureau of Fire (PBF) chiefs and inspectors can see which buildings in the city are at the highest risk of a fire incident and prioritize safety inspections accordingly.
A team at Carnegie Mellon University developed the Fire Risk Analysis model that uses machine learning to predict the likelihood of a fire occurring at a given property. Implemented in February 2018 and re-evaluated six months later, the CMU model found that in that six-month window, there were about 45 fire incidents, one-third of which occurred in buildings the model ranked as high-risk.
“What that tells us and the fire inspectors also is that they should be using this set of high-risk properties to inform where they’re inspecting,” said Michael Madaio, a graduate-research assistant at CMU’s Human-Computer Interaction Institute who runs the project.
The fire chief and inspectors had data from checking properties’ adherence to safety codes. They also had data on fires and where incidents were occurring “but they didn’t quite know whether they were targeting the properties that needed those inspections most.” he said.
To build the model, Madaio started with about eight years of historical data on fire incidents and property assessments at 15,000 commercial buildings that he obtained from PBF and other city agencies through a memo of understanding between the bureau and the university’s Metro21 Smart Cities Institute, which serves as a sort of laboratory for smart city projects. He also worked with Pittsburgh’s Department of Innovation and Performance, which coordinates data sharing across agencies.
Next, he and the team determined which dataset would be the most informative for predictions and worked to get the data in a consistent format. For instance, some city agencies used street addresses to identify properties, while others used parcels, so he combined and adjusted the data to work with addresses because that’s what PBF used for incident reporting.
Once the data was in a usable format, he turned to machine learning -- specifically, supervised learning. He fed six-to-seven years’ worth of data into the model as training data, from which it learned which attributes are associated with the event to be predicted. He used data from the eighth year to test how well the system could identify how likely a particular address was to have a fire incident within a particular time window, say, within the next six to 12 months.
“What we can get from the analysis is which features are most predictive overall,” Madaio said. “What the model can’t do is say for a particular property which attributes or which features are the most predictive.”
The model generates a likelihood of an event occurring on a scale of 1 to 10, and the results are integrated into the city’s existing open data platform called Burgh’s Eye View.
“It’s an interactive map dashboard. Various city agencies already use that for things like monitoring 311 calls or other alerts and city data. That was something that the fire department was already using, so we integrated these property risk scores into that existing interactive map,” he said.
Chiefs and inspectors can filter results on the map by property usage such as high-rise apartments or hospitals. When they zoom out, the map clusters high-risk properties together with a number, so users can see at a glance that a particular neighborhood has, for example, 35 high-risk properties. That way, if an inspectors are at one building, they can check the map to see if a high-risk property is nearby and swing by it, too.
Similarly, the dashboard also lets users filter by property type, neighborhood and fire district.
The fire risk prediction model is currently deployed to PBF’s server, where it reruns every week, generating new risk scores based on the most up-to-date fire and property data.
“We did not want to replace the inspectors’ decision-making,” Madaio said. “This should be just another tool in their toolkit.”
“It’s that combination of open data sources plus internal documents,” said Karen Lightman, executive director of Metro21. “Oftentimes, things can be stuck on someone’s laptop in an Excel spreadsheet or a PDF or something,” she said. “It’s a great example of how simply it can be done.... [Madaio] took the time to explore this issue and find ways of optimizing and improving the efficiency.”
Madaio started the fire risk analysis work with the Atlanta Fire Rescue Department before becoming a Ph.D. candidate at CMU. He helped create the Firebird framework, a similar model that computes fire risk scores for about 5,000 buildings with true positive rates of up to 71%, according to a report.