How automation can transform storage provisioning
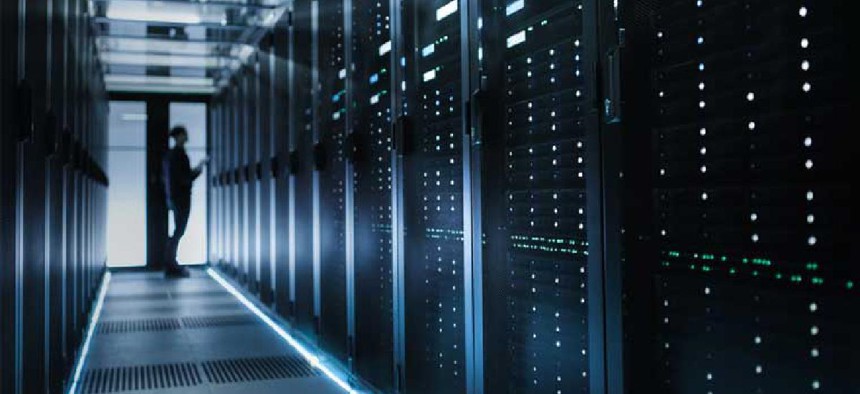
Automation provides the only practical path to effective data provisioning that can increase data center efficiency while ensuring users have the resources they need.
The diversity of systems and software running business operations has increased to the point where managing all the components can consume most of a data center teams’ energy, taking precious time away from planning and implementing new initiatives that move an agency’s mission forward.
To ease the burden on team bandwidth, data center leaders frequently rely on monitoring software that continually checks for system faults and outages so operations don’t fail. That’s useful for meeting uptime and data protection service level agreements (SLAs). However, it still doesn’t provide real-time monitoring that can ensure systems and software are optimized both individually and holistically. That optimization should cover adjusting applications, hypervisor, servers, networks and storage for peak performance and uptime.
Increasingly powerful artificial intelligence (AI) and machine learning (ML) technologies can instead allow the data center to monitor itself in real time, allowing leaders to focus more resources on strategic, forward-looking initiatives.
Predictive and prescriptive analysis pave the way
In an environment that is never static, AI- and ML-enabled systems can make decisions faster, more efficient and error free. It’s an ongoing process that requires predicting, prescribing and executing on specific actions. Predictive analysis depends on root cause analysis, where collected data is used to derive potential future outcomes. Prescriptive analysis takes that data to a deeper level to show potential results and enable corrective action before anything actually happens.
Both are enabled by two core machine learning approaches:
- Decision-tree learning, in which AI examines device status, uses logic to predict what will happen next, then prescribes appropriate configuration changes.
- Association-rules learning, in which information from devices is correlated to identify and prescribe changes to reach desired outcomes, for instance the best deployment model or root cause analysis.
Together, they provide the foundation of an autonomous data center, where day-to-day operations are managed by intelligent systems and software.
Automation supports provisioning decisions
One of the biggest opportunities for improving data center efficiency lies in automating common configuration and administrative tasks like storage provisioning. Storage administrators are saddled with repetitive, manual tasks that become increasingly complex from continually fluctuating capacity demands. Resource management can’t be based on static assessments, such as always allocating additional capacity from a specific array. Not only is that inefficient, it leaves room for more errors when administrators need to make quick decisions to satisfy user needs. What’s more, skillfully managing provisioning decisions often requires senior-level staff with deeper experience -- which is not the best use of their time.
Rather, decisions should be made based on the best option at any given time. Automation offers the opportunity for smart storage provisioning -- for example, analyzing storage telemetry data and using it to prescribe the best available resource to meet a goal or SLA. Using a programmable model will allow the decision map and outcomes to be adjusted as needed. The same goes for determining the most appropriate data path and switching zoning configurations when, for instance, deploying a new application.
Analysis informs strategic planning
Telemetry data from the application or hypervisor level, the network level and the actual storage level is needed to analyze and monitor the full data pipeline. By collecting shared or known instances and comparing them against unique SLAs, environmental variables can be customized for each unique data center situation. The insights can be used to make decisions based on the best outcomes, like prescribing the most appropriate data path across an entire infrastructure to maximize performance.
As tasks are repeated, ML enables more accurate suggestions on how to improve provisioning throughout the environment. Over time, the technology can inform critical strategic planning requirements: forecasting capacity, budget and performance; analyzing storage capacity and utilization trends over time; determining when resources will hit peak performance; managing performance thresholds; and predicting and automatically adjusting resource performance settings critical to the customer experience.
Ultimately, this automation improves overall efficiencies by optimizing an agency team’s time and talents, reducing errors and keeping users efficient and productive.
As complexity increases and business pressures mount, many data center leaders are starting to look towards AI and ML as important tools to transform their operations. Automation provides the only practical path to effective data provisioning that can support tomorrow’s requirements. Increasing data center efficiency while ensuring users have the resources they need smooths the way to the entire agency meeting its goals.