From terabytes to exabytes: Supporting AI and ML with object storage
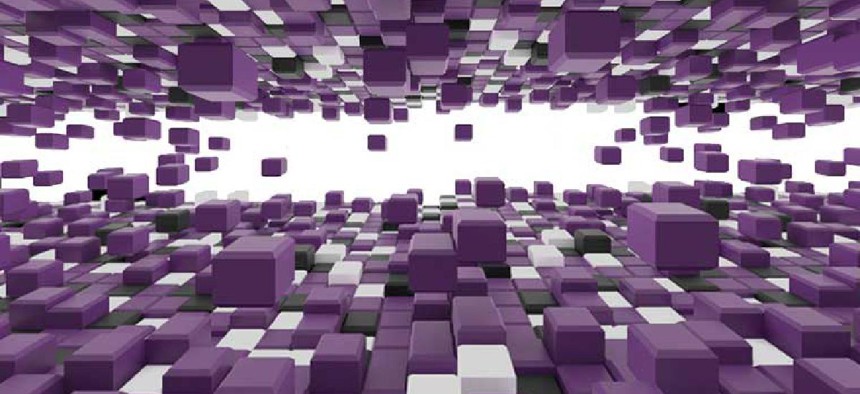
Connecting state and local government leaders
While object storage is a common solution for cold storage, recent advances make it ideal for artificial intelligence applications, which require higher performance storage.
From agriculture to defense, federal agencies are increasingly using artificial intelligence and machine learning to enhance mission-critical capabilities, accelerate research breakthroughs and free up staff resources.
The byproduct of this adoption is a rapidly increasing store of unstructured data in the form of images and video footage. The amount of unstructured data produced globally is growing by up to 60% per year and is projected to comprise 80% of all data on the planet by 2025, according to IDC.
All this data must be processed, analyzed, moved and stored. Currently, many organizations do this work using public cloud services. However, as the federal government continues to implement AI and ML technologies, many IT leaders are looking for a solution that better suits their needs in terms of cost, convenience and security.
Object storage -- which allows organizations to build their own private cloud storage environment on-premise, as well as unlocking edge computing capabilities -- is quickly emerging as a viable alternative.
So how does object storage work? How do different object stores compare to each other and the public cloud? And more importantly, how easy is it to implement and use? Read on to find out.
First things first
Object storage is a completely different approach to storage, where data is managed and manipulated into individual units called “objects.”
To create an object, data is combined with relevant metadata, and a custom identifier is attached. Since each object has comprehensive metadata, object storage removes the need for a tiered structure like the one used in file storage. It’s therefore possible to consolidate vast amounts of unstructured data into a single, flat, easily managed “data lake.”
Object storage is a common solution for cold storage archiving. However, with recent technological advances, data can now be accessed much faster, making it ideal for applications like AI and ML, which require higher performance storage.
Object storage vs. public cloud
The emergence of edge computing goes hand in hand with the rise of AI and ML. Using public cloud services to analyze and store data captured by internet-of-things devices and sensors works brilliantly in urban centers. However, from agricultural drones to bomb disposal robots, connectivity to a central cloud repository is likely to be significantly slower in areas with less-dense network infrastructure.
Object stores solve this problem with low-cost, remote storage that enables computing to happen at the edge. Processing data at the point of collection is significantly faster than sending everything into the cloud, where it must be processed and returned.
Additionally, much of the data used to train AI algorithms has to be stored long term for auditing purposes, another area in which object storage excels. Capabilities including versioning, end-to-end encryption, object locking, and ongoing monitoring and repair enable data to be preserved for decades at a much lower cost than in the public cloud.
Comparing different object stores
When weighing object storage options, it’s important to scrutinize the technical features of various products. For instance, some object stores make multiple copies of each object to protect against data loss, which can eat up storage very quickly.
On the other hand, more advanced object stores take advantage of erasure coding, which breaks up a unit of data and stores the fragments across various physical drives. If data is wiped or becomes corrupted -- whether by accident or because of malicious activity -- it can be reconstructed from the fragments stored on the other drives. This lowers storage costs, as it doesn’t require organizations to keep multiple copies of each object.
Plus, erasure-coded platforms can achieve incredible data durability, keep disk overheads low and enhance the overall performance of the system. Of course, not all vendors implement erasure coding the same way. Different products will likely have differing scalability, as well as varying rebuild and rebalance times.
Another important feature to examine is the data consistency model used by different object stores. “Strong consistency” is preferable for AI and ML applications. In short, this means that after a successful write, overwrite or deletion, any subsequent read request immediately receives the latest version of the object. Some object stores still use “eventual consistency,” where there’s a lag until read operations return the updated data. This means that the application will occasionally operate off older versions of the objects.
How easy is it to implement and use?
Ease of use is subjective, of course. However, object storage does have several advantages. For instance, it requires less day-to-day attention than a traditional storage-area network, since the resilience of the system allows multiple disks to fail without incurring data loss. This means over 200 petabytes can be managed by a single administrator.
There’s no doubt that managing data captured by AI and ML applications will continue to challenge government IT teams. Object storage is not a panacea, but does address cost, speed and security issues. Looking forward, agencies that adopt object storage should focus on implementing modular end-to-end data management solutions. These enable elements to be swapped out for more advanced technologies when they become available.