Overcoming Roadblocks to Evidence-Based Policy Making
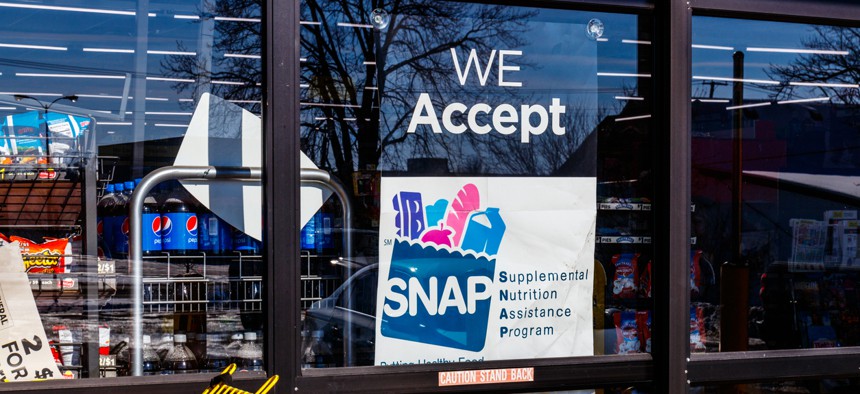
Interoperability of data across programs like SNAP, Medicaid and unemployment continues to be a chief data sharing challenge. iStock.com/jetcityimage
COMMENTARY | Promising initiatives like the California Policy Lab show how governments can take greater advantage of data.
Data are the key to unlocking solutions to our world’s most urgent social policy challenges. Take the Covid-19 pandemic. Data have played an important role in helping to understand and address issues like racial and ethnic disparities, as well as examining infection spread in schools.
In recent years, there has been a flurry of activity to indicate that federal decision makers intend to increase reliance on evidence to drive policies and programs. For example, the 2019 Foundations for Evidence-Based Policymaking Act (Evidence Act), President Biden’s executive order on evidence-based policymaking, the creation of the federal Advisory Committee on Data for Evidence Building, as well as the proposed task force on scientific integrity and National Secure Data Service all demonstrate a serious commitment to data driven decision-making. The same can be said of state governments, which have already implemented many foundational elements of the Evidence Act, including directing agencies to create learning agendas, appointing chief data officers, and conducting periodic capacity assessments.
Despite these advances, federal, state and local governments face complex challenges in using data to build a stronger foundation for evidence-based policy.
For example, data sharing—whether within states, between states or between states and the federal government—is a critical challenge that must be addressed in order to capitalize on the promise of evidence-based policy. In most cases, it is difficult (or even impossible) to look across Medicaid, Supplemental Nutrition Assistance Program, unemployment and education data, that all may impact the same individual or family. Some states have made progress toward improving interoperability of data across programs and agencies. The California Policy Lab, for example, partners with state and local governments to generate data and evidence that can be used to address homelessness, poverty and education inequities. This framework, which is based on conducting empirical research to improve program outcomes and help interconnected systems work more efficiently and effectively, can be applied in other states interested in sharing data and improving programs.
Program administrators also need high quality data to accurately forecast and manage costs, track access to services, monitor performance and deter fraud. Some states, including North Carolina, are making headlines for using administrative data to improve health care and reduce costs. But many state and local agencies lack the infrastructure, processes and human resources needed to collect, manage and validate data effectively. These states require services and supports that:
1. Boost investments in staff
2. Increase development and use of learning agendas
3. Provide states with robust technical assistance
4. Establish clear lines of communication between states and the federal government
My Mathematica colleagues have observed several instances where collaborative work between federal, state and local authorities can inform decisions. For example, through the Medicaid and Children’s Health Insurance Program (CHIP) Business Information and Solutions initiative, the Centers for Medicare & Medicaid Services is building an infrastructure for robust data analytics that integrates federal and state data sources to inform decisions about Medicaid and CHIP policy and programs. This partnership has resulted in a variety of tools for advanced data quality detection and dissemination. These tools are used to support technical assistance to states and include dashboards, exploratory analytics and custom reporting capabilities for self-service decision making.
We’ve also seen the Administration for Children and Families (ACF) at the U.S. Department of Health and Human Services establish the Division of Data and Improvement (DDI). This division is the lead on strategic planning, performance measurement, data security and privacy, and application of data for the continuous improvement of ACF-sponsored programs. DDI’s work also extends to supporting states and localities as they organize their data systems and data routines in support of program improvement.
While these models are promising, funding for community services and supports have declined steeply due to the economic fallout of the pandemic. At the same time, essential health, mental health and community services are being redirected to respond to the Covid-19 pandemic, which is increasing rates of chronic illness, injury, disability and mortality. Additionally, states are struggling to maintain pre-pandemic levels of services and supports, which limits deeper investments in data collection, security and analysis. While the recently signed American Rescue Plan will provide an influx of aid to states that should begin to alleviate these issues, more permanent solutions are necessary in the long run.
One way to address this complex issue is to co-create state and federal policy simulation models that enable us to forecast and compare the potential impact of various policy strategies on future rates of disability, illness and death. Such simulations are identifying insights to help assess and manage Covid-19’s impacts on communities.
Harnessing the power of data and evidence will enable policy leaders to make informed program improvements and better decisions. To do that, we must break down the existing barriers facing data collection and analyses across states while also building stronger support systems and infrastructure to facilitate increased reliance on data to address urgent social challenges.
Paul Decker is a nationally recognized expert in policy research, data analytics, education, and labor policy. As president and CEO of Mathematica, Decker sets the company’s vision and strategy, oversees its operation and management and shapes its values and standards.
NEXT STORY: Welcome to the New Progressive Era