How AI helps one city spot damaged rooftops
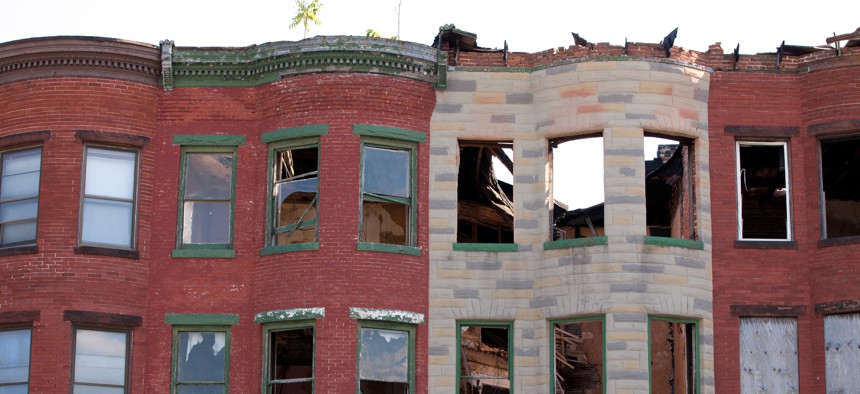
hroe/Getty Images
The Baltimore Department of Housing and Community Department is using a mix of artificial intelligence, GIS-based flyover imagery and building code enforcement data to automatically detect at-risk buildings.
To improve building maintenance and worker safety, the Baltimore Department of Housing and Community Development is combining artificial intelligence and aerial imagery to find collapsing roofs around the city.
A mix of AI, GIS-based aerial flyover imagery and building code enforcement data will give officials a tool that automatically detects at-risk infrastructure, DHCD said in a Jan. 17 announcement. The tool goes beyond the ability to point out if a building is vacant—it can break down how many of those buildings have rooftop damage and how many of them are right next to occupied properties, Deputy Commissioner for Permits and Litigation Jason Hessler said in an interview.
With the data, officials can determine if it’s going to cost $40,000 or $70,000 to stabilize those structures and “go after the necessary funding to address that problem,” he said. It will allow the city to “not just eliminate the number of vacant buildings with roof damage, but also prevent those neighboring properties from becoming vacant,” he said.
There are more than 14,000 vacant buildings in Baltimore, according to Hessler, a growing issue as the city’s population has been declining since the 1950s. When one property becomes vacant, it can create a chain reaction until the majority of or even the whole block is empty, he said. As a result, the buildings are left to deteriorate, and the city may turn to demolition of the property rather than rehabilitation or stabilization, which can be costly and time consuming.
Abandoned sites also breed safety, fire or health hazards as the buildings are vulnerable to wildlife, illegal dumping and the effects of weather. This creates “uncomfortable” environments, depreciates property values for nearby homeowners and could endanger city staff that interact with the building, Hessler said.
To tackle the issue, in 2022 DHCD partnered with the Data Science for Social Good (DSSG) research group at Carnegie Mellon University and Baltimore’s Chief Data Officer to test AI algorithms that automate the roof damage identification process. Program fellows helped DHCD develop three models that detected buildings with collapsed or damaged roofs.
The first model identified dark pixels in an overhead image of a building where the pixels represented holes in the roof, said Henry Waldron, director of research and analytics for Baltimore. While the agency relies on photos to assess properties, Hessler said the image quality does not allow it to identify specific individuals or activities, so privacy concerns are eliminated.
The second model scanned DHCD’s tabular data such as building notices, inspection notes and other records and pulled words such as “collapsed,” “collapsing” or “vacant” to indicate a property’s state. The third model was a neural network that was trained with historical data from 2018. The data helped the algorithm determine what a damaged roof would look like now based on the older images, Waldron said.
While the models performed adequately on their own, “a combination of all three models [yields] the strongest result,” he said.
The AI model is currently maintained by the DSSG team, and the city is in the process of getting the code from the researchers and reviewing software and hardware requirements to get the system up and running, Waldron said. The agency will train the algorithm using recent roof condition data so the model can be run every year with new aerial imagery, he said.
Staff manually review the data outputs in case the AI model incorrectly flags a home’s solar panel or deck as a collapsed structure. When staff do come across an image containing a damaged roof, inspectors are sent to investigate and determine if emergency action is needed to make repairs.
DHCD is also exploring new ways to use the data that the model produces. For instance, staff can now spot concentrations of damage in certain areas of the city, compare roof conditions in different neighborhoods or identify if a deteriorated structure is located next to an occupied building, Waldron said.
“We'll be able to catch damaged roofs earlier on in the process when they're 5% or 10% damaged as opposed to 80% or 90% damaged, and that could make a big difference in the cost of rehab of the property [and] the damage that caused the neighbors,” Hessler said.