How Agencies Can Automate Data Extraction at Scale
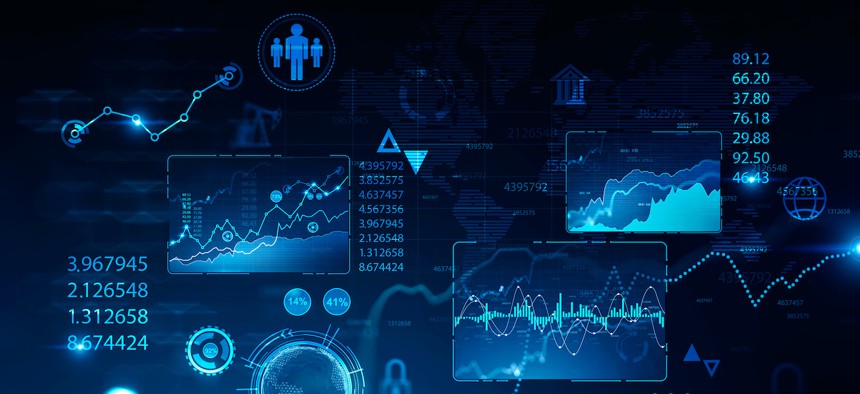
ismagilov/Getty Images
Connecting state and local government leaders
COMMENTARY | Data is embedded in formats that are hard to search and process. Think emails, PDFs, spreadsheets, images, driver’s licenses. Here's how to extract that data and improve efficiency, reduce backlogs and solve operational challenges.
State and local government agencies aspire to more fully leverage the vast volumes of data they collect and generate as strategic assets. After all, agencies that put data at a foundational core of their enterprise operation can dramatically advance their ability to make day-to-day management decisions, formulate policies, manage resources, and perform missions and business processes.
But leveraging data is far easier said than done. That’s because a large majority—by some industry estimates, 80% to 90%—of a typical enterprise’s data is embedded in formats that are hard to search and process. These formats include emails, PDFs, forms, images, spreadsheets, Word documents, maintenance logs, driver’s licenses and many others file types. The unstructured data in these files is not pre-formatted, making it difficult for agencies to take advantage of the information they have.
To search, access and use unstructured data, agencies often digitally scan the files and then have someone read through them, page by page, to find the data they need. Or, agencies can have in-house or contractor staff extract the needed data from these files and enter it by hand into business systems where it can then be easily searched, accessed and used. Entering this data manually into a business system is impractical for state and local government agencies given the large workloads they typically manage.
Automating manual data entry not only improves efficiency and cost, it also reduces backlogs and allows agencies to leverage their unstructured data more easily to solve operational challenges.
There are plenty of options for automatically extracting data from unstructured documents, so agencies should ensure the solution meets their requirements for accuracy and throughput in a production environment.
Data managers should be sure any solution has the versatility to take on more than a small number of projects without significant customization and expense. They should look for modular systems based on open architectures so the solution can easily incorporate new best-of-breed automation and artificial intelligence.
While optical character recognition technology can be used as a stand-alone solution, by itself it is insufficient to solve the manual review problem. It cannot automatically identify and extract pieces of information pertinent to a reviewer or an operator; nor can it identify pertinent sections or pages within a document. Template-based extraction, which combines OCR with software-defined rules, may also fall short because it quickly degrades when documents contain variability and requires a new template for each form.
A better approach for automating data extraction is to combine OCR with machine learning. It can handle highly structured forms as well as variability, processing completely unstructured documents such as reports with free-form text. Additionally, agencies can avoid ML models that require extensive training on thousands of samples by employing solutions that use pretrained, transformer-based models, which train on smaller datasets and can be deployed from low-code or no-code platforms.
Agencies should look for automated data extraction solutions that are modular, future-proof and scalable. Being modular gives the solution greater versatility and enables operators to easily reuse capabilities developed for one use case for other applications. Future-proofing means that a solution can be easily upgraded or enhanced with new, best-of-breed technologies as they emerge. It requires that the solution be designed around an open architecture, which helps ensure that the agency using it is not locked in to any particular vendor, technology or capability. Scalability simply means the solution can keep pace with the workloads of even the largest government agencies, which may process millions of documents a year.
When these capabilities are combined effectively, the benefits to the entire enterprise are truly unlimited. This technology, for example, allows government employees to translate text, identify entities and locations from free-form text documents, extract embedded images from within documents and even identify and extract handwritten values and signatures from unstructured documents.
In summary, the most capable approaches for automating data extraction today involve combining OCR and ML technologies. But there are many other factors that state and local government agencies should weigh as they do their market research to ensure that whatever solution they decide on is best suited for their needs.
Matt Macnak is director of public sector technical strategy at Instabase.
NEXT STORY: The Latest ‘Right to Repair’ Law Is the Broadest One Yet