AI pulls ahead in recidivism prediction
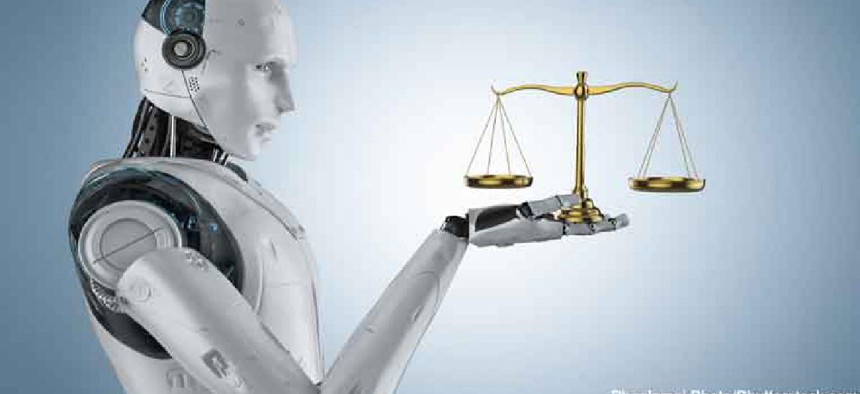
A new study finds that risk assessment tools are significantly better than humans at interpreting the complexity and noisy data of the criminal justice system.
Algorithms seem to be winning the latest round of the man vs. machine debate, at least when it comes to predicting recidivism.
A new study by researchers at Stanford University and the University of California at Berkeley found that risk assessment tools are significantly better at interpreting the complexity of the criminal justice system and making more accurate decisions than humans. When only a few variables are involved, humans can compete with sophisticated algorithms in predicting which defendants will later be arrested for a new crime. When a large number of factors are considered, however, the algorithms performed far better. In some tests, they were nearly 90% accurate in predicting which defendants might be rearrested; humans were on target only about 60% of the time.
“Although recent debate has raised important questions about algorithm-based tools, our research shows that in contexts resembling real criminal justice settings, risk assessments are often more accurate than human judgment in predicting recidivism,” said Jennifer Skeem, a psychologist who specializes in criminal justice at UC Berkeley and one of the authors of the study. “That’s consistent with a long line of research comparing humans to statistical tools,” she added.
Risk and assessment tools have been used to evaluate candidates for housing, financial services, insurance, health care and university admission, besides being tapped by the criminal justice system to inform decisions about bout bail, sentencing and parole.
Research conducted by Dartmouth University in 2018 cast doubt on commercially used risk-assessment software and algorithmic prediction. In that study, researchers recruited 400 volunteers with little or no criminal justice expertise to read vignettes that highlighted seven common risk factors about criminal defendants and asked them predict the likelihood of the defendants committing another crime within two years. The volunteers made correct assumptions about 63% of the time. Given the same vignettes highlighting the same seven features, the commercial software was right in 65% of the cases. At the time of the comparison, the software was capable of evaluating 137 factors.
Other studies also found problems. ProPublica cited the software’s racial bias, and researchers at Duke University built a simple algorithm that they said was as accurate as the commercial proprietary risk-assessment system.
What’s different about the new study, according to Berkeley News, is the number of factors evaluated and the experience of the volunteers.
Risk-related data is complex and noisy. Between pre-sentence investigation reports, victim impact statements and a defendant’s demeanor, humans are faced with “complex, inconsistent, risk-irrelevant and potentially biasing information,” the new study said. The researchers wondered whether more advanced risk assessment tools would be more effective than humans at predicting which criminals would re-offend when both are provided with more complex or otherwise noisy risk information.
To test the hypothesis, they expanded their research beyond the Dartmouth study, adding 10 more risk factors, including employment status, substance use and mental health. They also modified the experiment’s methodology from the Dartmouth study, not informing volunteers after each prediction whether their guesses were accurate.
As they suspected, on complex cases when volunteers were given more data points and didn’t have immediate feedback on their predictions to guide future decisions, they performed “consistently worse” than the commercial risk-assessment tool. The volunteers made correct recidivism predictions 60% of the time; the risk assessment tools was right in 89% of cases.
The performance difference “was not because the additional risk information compromised human judgment… [i]nstead, it was because models made better use of the additional information than did humans” the authors wrote.
The findings “support the claim that algorithmic risk assessments can often outperform human predictions of reoffending,” the researchers said, and they advocated for continued improvement of risk assessment algorithms, especially those that include seemingly irrelevant or potentially distracting information.
These tools play only a supporting role in the courtroom, Skeem noted. Ultimate authority rests with judges, probation officers, clinicians, parole commissioners and others who make decisions in the criminal justice system.
NEXT STORY: Library of Congress checks out 3D printing