Facial recognition: Can fake data produce real results?
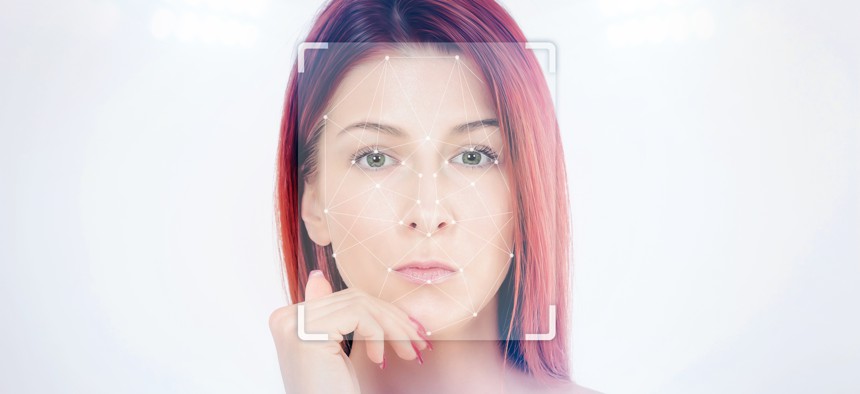
LumineImages/Getty Images
Diversifying training datasets with computer-generated faces may help break down bias in the technology, but one expert says using synthetic images is a step too far.
Artificially generated images may be the next step to combating bias in facial recognition software, according to researchers at the University of Southern California.
While often criticized for promoting discrimination or bias, facial recognition software’s accuracy has improved greatly over the years. The National Institute of Standards and Technology’s most recent facial recognition vendor test showed the top 150 algorithms were more than 99% accurate across various demographics.
However, inaccuracies can remain, especially when the source datasets used for training are biased themselves. When an image dataset underrepresents critical attributes such as gender, race, weight or even hair color, those features become susceptible to biased classifications, researchers Jiazhi Li and Wael Abd-Almageed wrote in a September study.
The researchers used machine learning models to create computer-generated images depicting less common features such as red hair or extra weight to train facial recognition programs. Upon conducting a fairness evaluation on the original dataset and the augmented dataset, researchers found that “synthetic images can achieve consistent performance with real data and further yields better fairness.”
One expert sees promise in using computer-generated faces as companies make strides to diversify training datasets. “Synthetic data … solves a lot of questions that people have—Do they have legal right to use this data? Is it a privacy issue?” said Daniel Castro, vice president of the Information Technology and Innovation Foundation.
“Getting more data is helpful, so synthetic data will likely be a cheaper alternative than collecting real data,” he said. As governments find more uses for facial recognition, such as scanning staffs’ faces to enter a secure building or using surveillance footage to identify witnesses and victims of a crime, officials should choose a vendor whose product is aligned with the agency’s specific use case, he said.
But others are skeptical of artificial images’ effect on facial recognition performance. “If the model is not trained with the right data, it will of course not come up with the right results,” said Jon Callas, the director of public interest technology at the Electronic Frontier Foundation, a nonprofit that advocates for civil liberties in the digital space.
Artificial images are likely to “make up” characteristics such as red hair, he said. As a result, a model may stick red hair on individuals that do not carry qualities typically associated with red hair such as pale skin and freckles, thus creating an image “that’s not really a red-hair person,” he said.
“You’re training [the] model on things that are not real people, and it will inevitably skew the model in directions we don’t know,” Callas said.
It's acceptable for some machine learning models to be wrong occasionally, Callas said, such as apps that generate images of what someone will look like when they’re much older. “If it’s only 90% accurate, that’s okay. But if it’s a law enforcement system, 90% accurate is not nearly good enough.”
NEXT STORY: Automated vehicles coming to Ohio roads